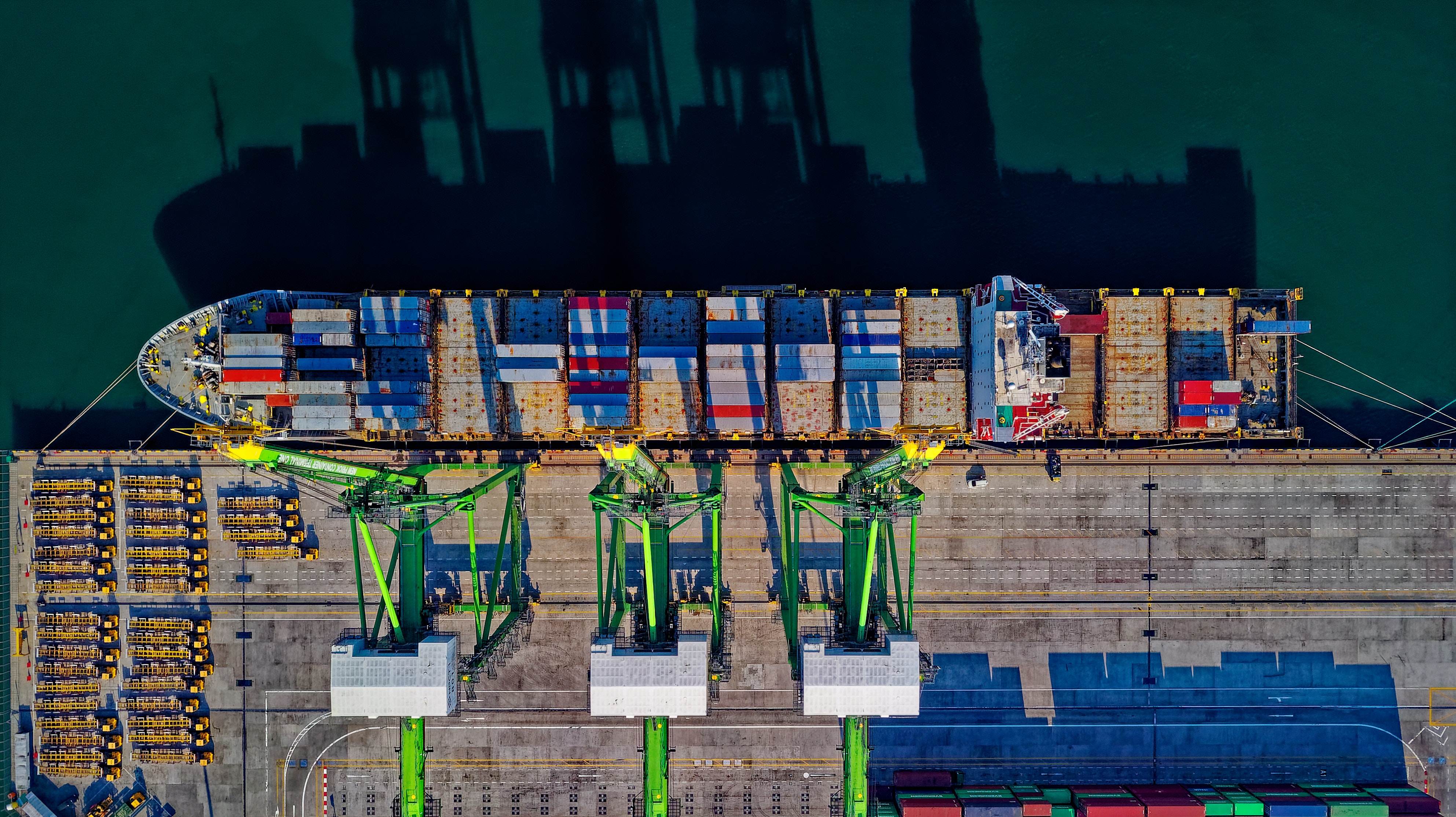
Data Science in Action: Revolutionizing Logistics for a Connected World
Introduction
In an era characterized by globalization, e-commerce, and rapid technological advancements, the logistics industry stands at the crossroads of unprecedented challenges and opportunities. Meeting the ever-growing demands of customers and ensuring the efficient movement of goods is no small feat. Fortunately, data science has emerged as a powerful ally, reshaping the logistics landscape in ways previously unimaginable. In this comprehensive exploration, we will delve into how data science is applied in the logistics sector, uncovering the remarkable transformations it brings to the transportation, tracking, and delivery of goods.
Predictive Analytics for Demand Forecasting
The heart of logistics beats to the rhythm of supply and demand. Accurate demand forecasting is the cornerstone of a well-functioning supply chain. Data science has revolutionized this critical aspect by harnessing the power of predictive analytics. Through the analysis of historical sales data, market trends, and external factors such as holidays and weather conditions, data scientists can create robust predictive models. These models provide organizations with invaluable insights into future demand patterns, enabling them to optimize inventory levels and allocation. The result is a finely-tuned supply chain that minimizes stockouts, reduces overstocking, and ultimately enhances customer satisfaction.
Route Optimization and Efficiency
Navigating the intricate network of roads, highways, and transportation hubs efficiently is a perpetual challenge in logistics. Enter data science, which has introduced a new level of precision and efficiency into route optimization. Advanced algorithms meticulously analyze a multitude of variables, including real-time traffic patterns, road conditions, vehicle capacities, and delivery schedules. By processing this wealth of data, logistics companies can determine the most efficient routes for their delivery vehicles. Not only does this save time and fuel costs, but it also contributes to environmental sustainability by reducing carbon emissions.
Real-Time Tracking and Visibility
Real-time tracking has become the norm in logistics, and data science is at the forefront of enabling this transformation. IoT (Internet of Things) devices, sensors, and GPS technology provide continuous, real-time data on the location, condition, and security of cargo. This information is accessible to logistics companies and their customers alike, ensuring transparency and accountability throughout the supply chain. Whether it's monitoring the temperature of perishable goods, tracking the progress of a package, or ensuring the security of high-value cargo, real-time tracking enhances the reliability and security of logistics operations.
Warehouse Management and Automation
Inside distribution centers and warehouses, data science is a driving force behind optimization and automation. Machine learning algorithms analyze historical sales data and inventory turnover rates to predict which products are likely to be in demand soon. Armed with these predictions, warehouses can rearrange their storage layouts to ensure that frequently requested items are readily accessible, reducing the time required to fulfill orders. Robotics and automation, guided by data-driven insights, are also becoming increasingly common. These technologies streamline order picking, packing, and shipping processes, not only accelerating order fulfillment but also minimizing errors.
Risk Management and Security
The logistics industry operates in a dynamic and sometimes unpredictable world. Data science plays a crucial role in assessing and mitigating risks. Predictive analytics, for instance, can identify potential disruptions such as severe weather events, labor strikes, or political instability that might affect supply chains. Armed with this foresight, companies can implement contingency plans and risk mitigation strategies to ensure the continuity of their operations. Furthermore, data science can enhance security measures by monitoring cargo in real-time, providing alerts for unauthorized access or tampering, and thereby safeguarding valuable shipments.
Customer Experience and Personalization
In the age of e-commerce, customer expectations have reached new heights, and logistics companies are leveraging data science to meet these demands. By analyzing customer data, including preferences, past behaviors, and location, logistics providers can offer personalized delivery options. These options might include same-day delivery, preferred delivery windows, or even the choice of a convenient pickup location. This level of personalization not only improves customer satisfaction but also fosters brand loyalty, as customers feel that their unique needs and preferences are valued and prioritized.
Conclusion: Data Science as the Catalyst for a Seamless Future
The application of data science in logistics is nothing short of revolutionary, ushering in an era characterized by unprecedented efficiency, transparency, and customer-centricity. From predictive analytics that optimize inventory levels to route optimization that reduces transportation costs, real-time tracking that ensures cargo security to warehouse automation that accelerates order fulfillment, data science permeates every aspect of the logistics industry.
In this fast-paced, interconnected world, where speed and reliability are paramount, data science has become an indispensable tool for logistics professionals. As technology continues to advance and more data becomes available, the logistics industry will continue to evolve, providing even more innovative solutions to meet the demands of our interconnected world. Data science is not just a tool; it's the compass guiding the logistics industry toward a future of seamless global supply chains, where goods flow efficiently, customers are delighted, and sustainability is prioritized.