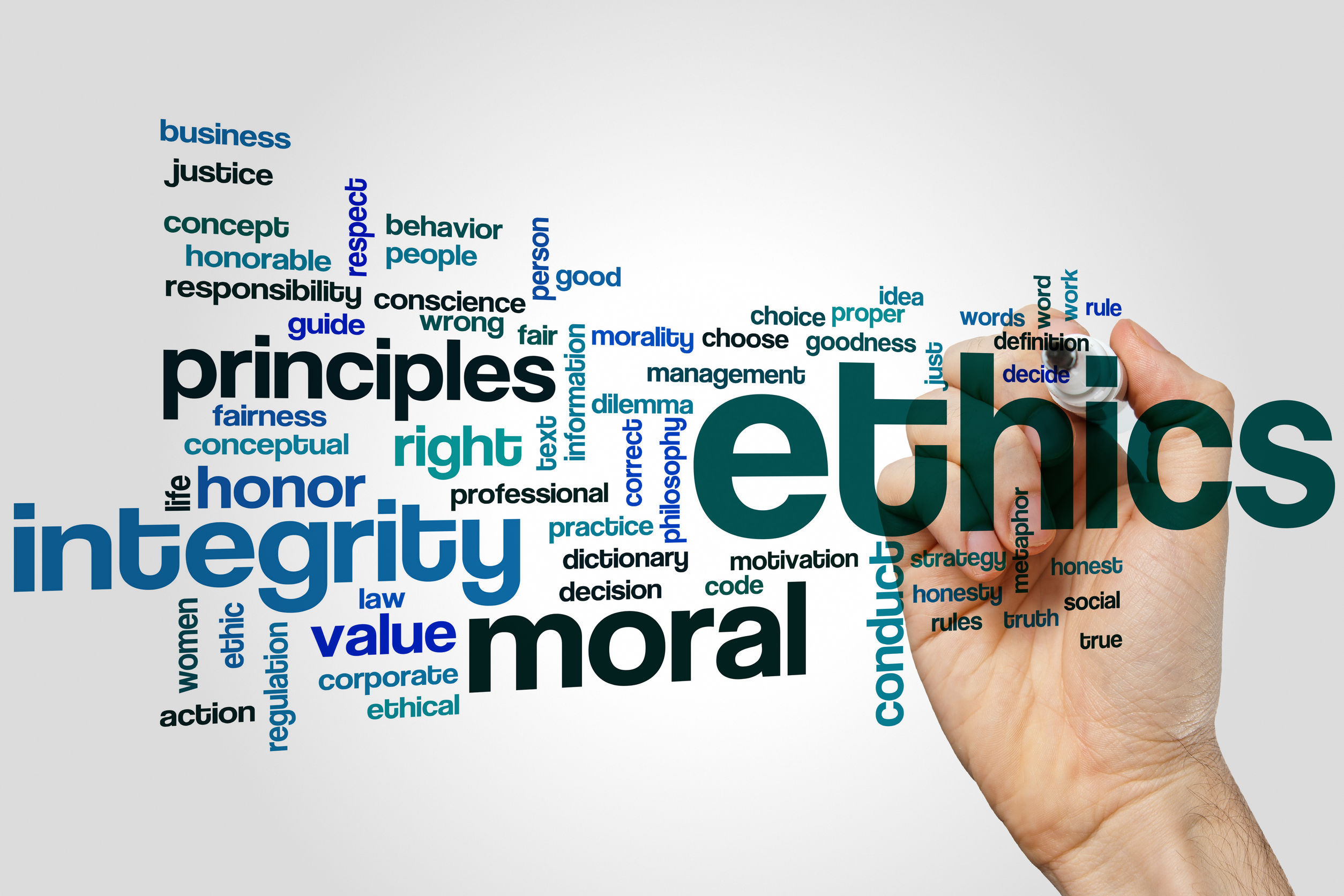
Ethical Considerations In Data Science: Navigating the Complex Landscape
Intrduction
In an era dominated by data-driven decision-making, the role of data scientists carries significant weight. Armed with the ability to extract profound insights from vast datasets, they empower organizations to fine-tune their operations, craft personalized user experiences, and forecast future trends with remarkable accuracy. Yet, this newfound power is inextricably intertwined with an immense responsibility. In the relentless pursuit of data-driven excellence, data scientists must adeptly navigate a multifaceted ethical landscape, one that demands unwavering attention to issues encompassing data privacy, bias mitigation, and the pursuit of fairness.
This blog post embarks on an exploratory journey into these pivotal ethical considerations that loom large in the data science domain. Within this intricate terrain, we will delve into the nuanced challenges data scientists encounter and unveil the innovative solutions they employ to uphold the mantle of responsible data usage. By the end of this discourse, I hope to shed light on the intricate web of ethics in data science, offering insights that empower data scientists to navigate these complexities with wisdom, integrity, and an unwavering commitment to the greater good.
Data Privacy: Respecting Individuals' Rights
Data privacy stands as a fundamental and unwavering pillar within the realm of ethical data science. At its core, it is an unyielding commitment to upholding the rights of individuals to exercise authority over their personal information. In this digital age, where data flows like a river, data scientists are entrusted with a profound responsibility: to be the guardians of sensitive data. This duty extends far beyond mere goodwill, encompassing a rigorous dedication to compliance with stringent data protection regulations such as the General Data Protection Regulation (GDPR) and the Health Insurance Portability and Accountability Act (HIPAA). These regulations serve as formidable guardians themselves, erecting a legal framework that demands meticulous care and stringent adherence from data scientists, ensuring that data is not just a commodity, but a safeguarded treasure of personal integrity and privacy. In this context, data scientists emerge as both custodians and stewards, playing a pivotal role in the ethical and responsible utilization of data in the modern world.
- Informed Consent: Always obtain informed consent when collecting personal data. Explain how the data will be used and provide individuals with the option to opt out.
- Data Anonymization: Anonymize or pseudonymize data to protect individuals' identities while still allowing for analysis.
- Data Security: Implement robust security measures to prevent data breaches and unauthorized access.
Bias in Data and Algorithms: The Fairness Challenge
Bias in data and algorithms is an intricate and pervasive challenge that permeates the very essence of data science. It is a multifaceted issue that can have profound implications for the fairness and equity of outcomes in various domains. The genesis of this predicament often lies in the data itself, as datasets can inadvertently reflect historical biases, prejudices, or societal inequalities. When such biased data is employed to train machine learning algorithms, it can perpetuate and even exacerbate these injustices, resulting in outcomes that discriminate against certain groups, reinforce existing disparities, and undermine the principles of fairness and justice.
Within this complex landscape, data scientists bear a profound responsibility. They must assume the role of vigilant guardians against the insidious influence of bias, diligently scrutinizing datasets for any signs of partiality or discrimination. This process demands meticulous data preprocessing, including identifying and rectifying imbalances and inaccuracies, and ensuring that data is representative of the diverse populations it affects.
Furthermore, the ethical duty of data scientists extends to algorithmic transparency and fairness. They must design algorithms that are not only accurate but also equitable, striving to eliminate any discriminatory patterns in the decision-making process. This involves continuous monitoring and refinement, as well as the implementation of mechanisms such as fairness-aware machine learning techniques to rectify and prevent bias in real-time.
In essence, addressing bias in data and algorithms is a paramount ethical imperative for data scientists. It underscores their commitment to the equitable and just application of data-driven technologies, promoting a future where data serves as a catalyst for positive change rather than perpetuating historical injustices. As the stewards of data and its transformative potential, data scientists play a pivotal role in shaping a more equitable and unbiased world.
- Data Bias: Recognize that historical data can contain biases, such as racial or gender biases. Be aware of how these biases might affect your models.
- Algorithmic Bias: Regularly assess your algorithms for bias and fairness. Adjust your models to reduce disparities in outcomes.
- Transparency: Make your data collection and model training processes transparent, allowing stakeholders to understand potential biases.
Fairness: Ensuring Equitable Outcomes
The concept of fairness lies at the very heart of responsible data science, intimately intertwined with the battle against bias. It embodies the ethical imperative to ensure that the fruits of data-driven endeavors, such as predictive models and algorithms, yield equitable outcomes for all individuals, irrespective of their diverse demographic characteristics.
To achieve this noble goal, data scientists must embark on a multifaceted journey. Firstly, they must conscientiously select and preprocess data, meticulously scrutinizing it for any traces of bias or discrimination. This involves not only rectifying existing imbalances but also fostering inclusivity by actively seeking diverse and representative datasets that encompass the broad spectrum of human experiences.
The pursuit of fairness extends to the design and training of machine learning models. Data scientists should adopt fairness-aware machine learning techniques that prioritize equity and prevent discriminatory decision-making. These approaches necessitate careful consideration of both the model's input features and the algorithm's optimization criteria, striving to avoid reinforcing or amplifying historical disparities.
Moreover, transparency is a cornerstone of fairness. Data scientists should be prepared to provide clear and comprehensible explanations for how their models arrive at particular decisions, ensuring that the black-box nature of some algorithms does not compromise accountability. This not only builds trust with stakeholders but also allows for the identification and rectification of any unintended bias that may arise during deployment.
In essence, fairness serves as the North Star guiding data scientists in their quest to harness the power of data for the betterment of society. By embracing fairness as a core tenet of their practice, data scientists can contribute to a future where data-driven technologies are not only accurate and effective but also instruments of justice, promoting a more equitable world where opportunities and outcomes are accessible to all.
- Fairness Metrics: Use fairness metrics to evaluate model performance across different subgroups. This helps identify and rectify disparities.
- Bias Mitigation Techniques: Employ bias mitigation techniques such as re-sampling, re-weighting, or adversarial debiasing to ensure fairness.
- Ongoing Monitoring: Continuously monitor model performance to detect and address fairness issues as they arise.
Accountability and Ethics Committees: A Collaborative Approach
Promoting ethical considerations within the realm of data science transcends the actions of individual practitioners. Instead, it calls for a holistic and organizational commitment, which can be realized through the establishment of ethics committees or data governance teams. These bodies serve as custodians of ethical principles, ensuring that they are not just lofty ideals but actionable guidelines that permeate every facet of an organization's data-driven endeavors.
The formation of ethics committees or data governance teams represents a proactive step towards cultivating a culture of ethical awareness within an organization. These committees consist of diverse experts, including data scientists, ethicists, legal professionals, and representatives from different departments, offering a broad spectrum of perspectives. Through regular meetings and collaborative efforts, they deliberate on the ethical implications of data-related projects, providing guidance and oversight to ensure that ethical considerations are integrated into decision-making processes.
Collaboration stands as the linchpin of these committees. By bringing together a diverse array of stakeholders, organizations can harness the collective wisdom and expertise required to navigate complex ethical dilemmas. This collaborative approach fosters a shared understanding of ethical best practices, enabling data scientists and other professionals to make informed decisions that align with the organization's ethical framework.
Moreover, ethics committees or data governance teams play a pivotal role in compliance with regulatory frameworks and industry standards. They keep abreast of evolving legal and ethical landscapes, helping organizations adapt their practices to remain in line with emerging requirements.
In conclusion, the establishment of ethics committees or data governance teams signifies a proactive and organizational commitment to ethical data science practices. Through collaboration and interdisciplinary dialogue, these bodies serve as guardians of ethical standards, ensuring that data-driven initiatives not only achieve their objectives but also adhere to principles that prioritize the well-being and rights of individuals and society at large.
- Ethics Committees: Encourage organizations to establish ethics committees composed of cross-functional teams, including data scientists, legal experts, and ethicists.
- Bias Mitigation Techniques: Develop clear ethical guidelines and policies to ensure that data science projects align with organizational values.
- Regular Training: Provide ongoing ethics training to data scientists and employees involved in data-related tasks.
Conclusion: The Ongoing Journey Towards Ethical Data Science
In the ever-evolving landscape of data science, ethical considerations stand as the lodestar that guides responsible practices. They serve as the foundation upon which the entire edifice of data-driven decision-making rests. Data scientists must adopt an unwavering commitment to ethical principles, recognizing that ethical dilemmas can surface at any juncture throughout the lifecycle of a project.
Central to this ethical framework is the preservation of data privacy. It is not merely a checkbox on a compliance list but a fundamental human right that must be upheld with utmost diligence. Data scientists must remain resolute in their efforts to protect personal information, thereby cultivating trust and safeguarding the sensitive data upon which they operate.
Bias, another formidable ethical challenge, must be confronted head-on. It is imperative to acknowledge that bias is insidious and can seep into data and algorithms, often unintentionally. However, data scientists must remain vigilant, continuously scrutinizing their datasets, and employing advanced techniques to identify and mitigate bias. In doing so, they endeavor to create a level playing field, reducing the risk of reinforcing existing inequalities.
Fairness, the linchpin of ethical data science, should be the guiding star that informs every decision. Data scientists are tasked with the mission of crafting models and algorithms that transcend demographic disparities, ensuring that the benefits of data-driven insights are accessible to all. This commitment to equity necessitates transparency, accountability, and a profound dedication to eradicating any form of discrimination from the data-driven decision-making process.
In conclusion, ethical data science is not a static goal but a dynamic and ongoing journey. It requires unwavering dedication to the highest ethical standards, with a willingness to adapt and evolve as new challenges and opportunities emerge. As data scientists, we are not just practitioners but custodians of a responsible and equitable data-driven future. In this complex and ever-changing landscape, our role is pivotal in shaping a better, more ethical, and more responsible world for all. It is a journey that demands constant reflection, adaptation, and a steadfast commitment to doing what is right for individuals and society as a whole.